Showing 5 results for Regression
B. Shahbazi, B. Rezai, S. Chehreh Chelgani, S. M. J. Koleini, M. Noaparast,
Volume 12, Issue 1 (3-2015)
Abstract
Multivariable regression and artificial neural network procedures were used to modeling of the input power
and gas holdup of flotation. The stepwise nonlinear equations have shown greater accuracy than linear ones where
they can predict input power, and gas holdup with the correlation coefficients of 0.79 thereby 0.51 in the linear, and
R2=0.88 versus 0.52 in the non linear, respectively. For increasing accuracy of predictions, Feed-forward artificial
neural network (FANN) was applied. FANNs with 2-2-5-5, and 2-2-3-2-2 arrangements, were capable to estimating of
the input power and gas holdup, respectively. They were achieved quite satisfactory correlations of 0.96 in testing stage
for input power prediction, and 0.64 for gas holdup prediction
A. Azizi, S.z. Shafaei, R. Rooki,
Volume 13, Issue 2 (6-2016)
Abstract
Nowadays steel balls wear is a major problem in mineral processing industries and forms a significant part of the grinding cost. Different factors are effective on balls wear. It is needed to find models which are capable to estimate wear rate from these factors. In this paper a back propagation neural network (BPNN) and multiple linear regression (MLR) method have been used to predict wear rate of steel balls using some significant parameters including, pH, solid content, throughout of grinding circuit, speed of mill, charge weight of balls and grinding time. The comparison between the predicted wear rates and the measured data resulted in the correlation coefficients (R), 0.977 and 0.955 for training and test data using BPNN model. However, the R values were 0.936 and 0.969 for training and test data by MLR method. In addition, the average absolute percent relative error (AAPE) obtained 2.79 and 4.18 for train and test data in BPNN model, respectively. Finally, Analysis of the predictions shows that the BPNN and MLR methods could be used with good engineering accuracy to directly predict the wear rate of steel balls.
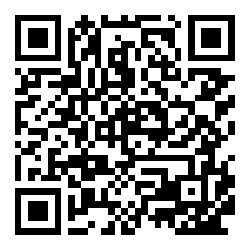
M. R. Ghaani, P. Marashi,
Volume 15, Issue 3 (9-2018)
Abstract
Na super ionic conductive (NASICON) materials are ceramics with three-dimensional scaffolds. In this study, Li1.4Al0.4Ti1.6(PO4)3 with NASICON structure was synthesized by Pechini method. As a result, a sample having a total conduction of 1.18×10-3 S cm-1 was attained. In addition, various parameters were studied to obtain high value of conductivity, by optimizing the process. The optimization was made using L16 Taguchi based orthogonal array, followed by ANOM, ANOVA and stepwise regression. As a result, the optimum synthesis parameters can be obtained, while pH of the solution was adjusted to 7. The ratio between the concentration of citric acid to metal ions and ethylene glycol concentration stuck to 1 and 2.5, respectively. The best heat treatment can be carried out with a combination of pyrolysis at 600 ºC and sintering at 1000 ºC.
E. Shahmohamadi, A. Mirhabibi, F. Golestanifard,
Volume 17, Issue 1 (3-2020)
Abstract
In the present study, a soft computing method namely the group method of data handling (GMDH) is applied to develop a new and efficient predictive model for prediction of conversion percentage of silicon. A comprehensive database is obtained from experimental studies in literature. Several effective parameters like time, temperature, nitrogen percentage, pellet size and silicon particle size are considered. The performance of the model is evaluated through statistical analysis. Moreover, the silicon nitridation was performed in 1573 k and results were evaluated against model results for validation of the model. Furthermore, the performance and efficiency of the GMDH model is confirmed against the two most common analytical models. The most effective parameters in estimating the conversion percentage are determined through sensitivity analysis based on the Gamma Test. Finally, the robustness of the developed model is verified through parametric analysis.
Imtiaz Ali Soomro, Srinivasa Rao Pedapati, Mokhtar Awang, Afzal Ahmed Soomro, Mohammad Azad Alam, Bilawal Ahmed Bhayo,
Volume 19, Issue 4 (12-2022)
Abstract
This paper investigated the optimization, modelling and effect of welding parameters on the tensile shear load bearing capacity of double pulse resistance spot welded DP590 steel. Optimization of welding parameters was performed using the Taguchi design of experiment method. A relationship between input welding paramaters i.e., second pulse welding current, second pulse welding current time and first pulse holding time and output response i.e, tensile shear peak load was established using regression and neural network. Results showed that maximum average tensile shear peak load of 26.47 was achieved at optimum welding parameters i.e., second pulse welding current of 7.5 kA, second pulse welding time of 560 ms and first pulse holding time of 400 ms. It was also found that the ANN model predicted the tensile shear load with higher accuracy than the regression model.